Introduction
The difficulty in ensuring software security and the frequency of hacking incidents underline the need for workable solutions. There is a rising need for creative ways to deal with these issues as cyber attacks become more sophisticated and prevalent. Software security can potentially be improved with the use of Artificial Intelligence (AI). AI is a valuable tool for strengthening software security because it can analyze data, spot patterns, and identify potential dangers in real-time. To properly incorporate any new technology into a complete security strategy, it's crucial to grasp both its strengths and limitations. This essay will examine how AI might enhance software security, its drawbacks, and the need of a comprehensive strategy for software security.
How AI Can Improve Software Security
AI can significantly improve software security by quickly identifying and thwarting assaults. Predictive modeling and other AI-based techniques for anomaly or intrusion detection are used to achieve this. Analyzing system behavior and spotting odd patterns that can point to an attack is known as anomaly detection. Machine learning methods are used in intrusion detection to find well-known attack patterns and stop them from doing damage. On the other side, predictive modeling makes use of previous data to anticipate potential hazards and actively counteract them.IBM and Microsoft are two well-known businesses that have effectively applied AI to enhance their software security. IBM uses threat detection and response systems that are AI-based, and Microsoft uses AI-based predictive modeling to find vulnerabilities before they can be exploited. These illustrations show how AI has the ability to improve software security and defend against online threats.
Limitations of AI in Making Software Unhackable
Despite the possibility that AI could enhance software security, it's critical to recognize its limitations. AI cannot ensure total security and cannot provide a complete solution to making software unhackable. With AI-based systems, false positives and negatives are a common problem that can cause normal operations to be classified as malicious or the opposite. However, human control and involvement are still necessary for AI systems to operate accurately and effectively. However, it's possible that AI-based systems could be breached or manipulated, creating security holes in software. As a result, even though AI has a significant impact on software security, this impact should be viewed in the context of a bigger, more comprehensive security strategy that takes into account a variety of aspects, including personnel training, program design, and routine upgrades.
Threats to Consider with AI-Based Software
AI-based software is not impervious to dangers and weaknesses, as is the case with all technologies. It's important to note that AI can be influenced or hacked. AI system flaws can be used by attackers to get around security and access private information. AI-based systems may also generate false positives or false negatives, resulting in security holes or pointless alerts. AI systems may also be biased, which occurs when the system generates unfair or discriminatory conclusions as a result of the data it was trained on. It's crucial to put appropriate security measures in place and often upgrade AI systems to fix any known flaws in order to counteract these dangers. The possibility of bias can also be reduced by making sure AI decision-making is transparent and equitable. Companies may guarantee the ongoing security of their systems and data by identifying and resolving the potential vulnerabilities posed by AI-based software.
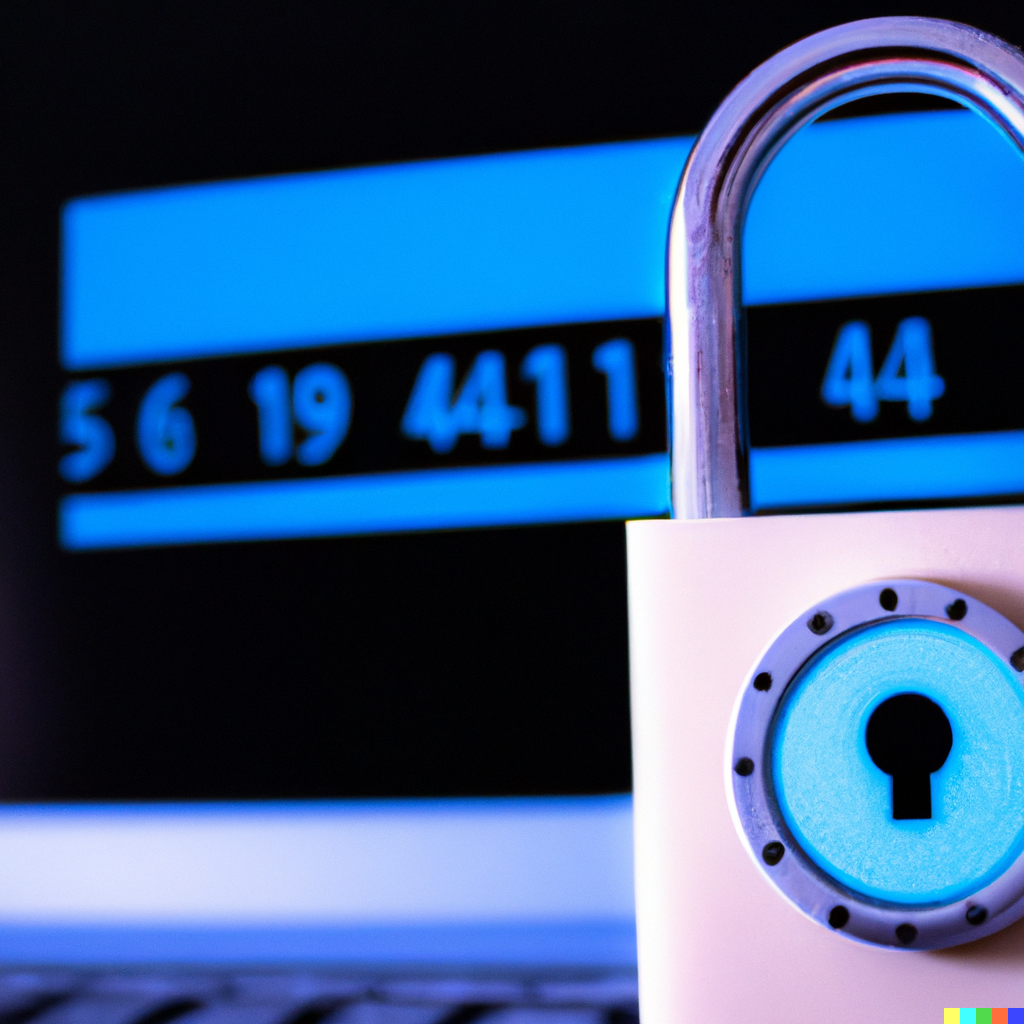
The Importance of a Holistic Approach to Software Security
A holistic strategy that incorporates AI as one of several components is necessary to provide complete software security. Several aspects, such as employee training, program design, and routine upgrades, all have an impact on the security of software. These aspects should all be addressed in a comprehensive security policy. This entails training staff members about security best practices, such as password management and phishing awareness, as well as developing software with security in mind to obviate vulnerabilities and updating it frequently to fix known flaws. Companies can reduce their vulnerability to cyberattacks and guard against the compromise of critical data by adopting a comprehensive strategy. For instance, Google has a thorough security policy that includes multi-factor authentication, employee training, and routine software updates, which has assisted the business in preventing numerous high-profile attacks. Companies can keep ahead of changing cyberthreats and defend their data and systems from potential attacks by integrating AI with a thorough security strategy.
Conclusion
In conclusion, while AI has the potential to improve software security, it's critical to understand that it isn't a panacea. Software security requires a complete security strategy with a number of elements, including personnel training, program design, routine upgrades, and AI-based solutions. Companies can reduce their vulnerability to cyberattacks and guard against the compromise of critical data by adopting a comprehensive strategy. AI-based systems can identify and stop threats in real time, but they are not infallible and still need human supervision and intervention. Hence, rather than being considered a stand-alone solution, AI should be seen as a part of a bigger security strategy. Companies may improve their software security and keep up with new cyber threats by taking a comprehensive approach.
Do you want to get to know how to make the most of AI and GPT-like models? Check our last article here!