In this article we start our series of articles that form the Algorand course for beginners. From the series of articles you will learn, among other things, how to set up a development environment for Algorand, how to deploy a smart contract on the Algorand network of your choice, how to write a simple smart contract, and what tools and frameworks you can use to work with the Algorand blockchain.
Algorand is a "green blockchain" launched in 2019, with the overarching goal of solving the blockchain trilemma through transaction speed, security, and a consensus algorithm that ensures full decentralization of the network.
A list of the necessary tools and components:
- Visual Studio Code
- Python 3.6 or later
- pyTeal library and py-teal-sdk
- Docker Desktop
- Algorand Sandbox
- Skeleton project repository
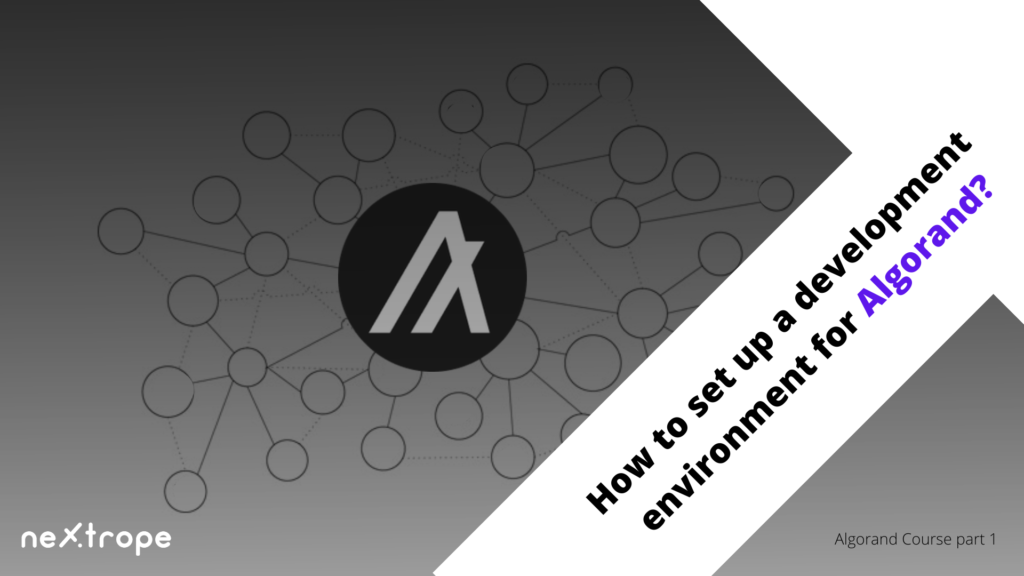
Visual Studio Code installation
Visual Studio Code will be your IDE, with the help of this program you will be able to write application code.
Visual Studio Code (known as VS Code) is a free and open source text editor from Microsoft. VS Code is available for operating systems: Windows, Linux and macOS. Although the editor is relatively lightweight, it includes several advanced features that have made VS Code one of the most popular development environment tools in recent times.
- Go to https://code.visualstudio.com/download and download the installation file compatible with your operating system.
- Install Visual Studio Code on your computer and proceed to the next step in this tutorial.
Installing Python
Python is a general-purpose, high-level interpreted programming language commonly used for web development, data analysis and automation.
One way to write smart contract logic for the Algorand network is with the Python library pyTeal ( https://pyteal.readthedocs.io/en/stable/ ), which allows you to write smart contract logic in python and compile the code into the TEAL code required by the AVM (Algorand Virtual Machine).
- TEAL is an assembly language syntax for specifying a program that is eventually converted to AVM (Algorand Virtual Machine) byte code.
- In VS Code, go to the "Extensions" tab used to install add-ons for Our IDE.
In the search bar, type "Python" and install Python extension for VS Code.
The next step is to install the interpreter for Python. This process varies depending on the operating system you are using.
Windows:
- Download the installer for the language version of your choice from the official Python website https://www.python.org/downloads/ then go through the standard installation process,
- An alternative to the above method is to install Python from the Microsoft Store, all the latest versions of python are available there.
MacOS:
- To install the Python interpreter on macOS, we need to use the Homebrew package manager,
- If Homebrew is already installed, open the command line and enter the command
brew install python3
.
On Linux distributions, the Python 3.x interpreter is installed by default.
After installing Python, it's a good idea to check that everything went as expected. To see the currently installed version of the interpreter, enter the command at the command line
python -v
Installing Docker Desktop
Docker Desktop is an easy-to-install application for macOS, Linux and Windows environments that allows you to create and share containerized applications and microservices.
Docker Desktop is required by the Algorand sandbox, a toolkit provided by the Algorand developers that is, a must-have, for any Algorand developer no matter what his or her level of expertise. Without Docker Desktop, you won't be able to run the most important tool for Algorand developers.
Installation files for each environment are available here.
After installation, Docker Desktop will start automatically.
Downloading Algorand Sandbox
Algorand sandbox is a set of tools that facilitate communication and interaction with the Algorand blockchain. Components of the sandbox include indexer, goal and algod.
With sandbox you can run betanet, testnet and mainnet in network mode, create tokens, nodes, execute transactions, create wallet addresses, check account balances, or deploy your applications on the network of your choice.
- Go to https://github.com/algorand/sandbox and copy the link to the sandbox repository
- Then open the command line on your computer, navigate to the desired location and enter the command
git clone <repository address>
- The sandbox repository will be cloned to your computer and you can start using it right away
Downloading the repository skeleton
To get started with Algorand easily enough, you should use the project skeleton, prepared by the Algorand development team.
- Go to https://github.com/algorand-devrel/pyteal-course and copy the link to the repository
- Analogous to the Algorand Sandbox download, go to the desired location and clone the repository to your computer
Running Python (venv)
Python venv is a virtual Python environment in which the Python interpreter, libraries and scripts installed in it are isolated from those installed in other virtual environments and (by default) any libraries installed in "system" Python, i.e. one that is installed as part of the operating system.
To run a virtual Python environment follow a few simple steps:
- Open the previously downloaded project skeleton in VS Code and start the terminal,
- Make sure you are in the root directory of the project and enter the command
python -m venv venv
, the python virtual environment will be created with the name "venv", - Then depending on the operating system you are using, enter another command to activate the virtual environment:
- MacOS:
source ./venv/bin/activate
, - Windows: source ./venv/Scripts/activate,
- To make sure you are working on the correct virtual environment, check that the name of your virtual environment appears before the command line in the terminal.
Installing additional libraries
In the example project in the requirements.txt folder, there are additional libraries that you need to install to start writing code for your application using the pyTeal library.
PyTeal is a Python language library for constructing Algorand smart contracts. It was created as a community project. The main goal of this library is to make writing contracts even easier and more accessible for programmers who prefer programming in Python.
- To install additional libraries into your virtual environment you need to put is in the project's root directory in the requirements.txt file,
- The most important libraries that we will use when writing smart contracts for Algorand are pyTeal and py-teal-sdk,
- Once you have the requirements.txt file enter the following command to start installing additional libraries,
pip install -r ./requirements.txt
.
Linking your project to a sandbox
When creating your blockchain application, after some time you may need, for example, to deploy it on a network of your choice. For this task you will need the Algorand sandbox, but first you need to properly connect your project to the sandbox by pointing it to the location of Our Project, among other things.
To do this, navigate to the folder where the Algorand sandbox you downloaded earlier is located and follow the instructions below:
- Open the docker-compose.yml file and in the services.algod section enter the additional volumes key with three additional parameters:
- -type: bind,
- source: <path to your project>,
- target: /data,
- example in the graphic below:
Starting the Algorand sandbox
To interact with the Algorand blockchain using the sandbox, you must first launch the sandbox container in Docker Desktop application.
To do so, follow the instructions below:
Open the command line on your computer and navigate to the folder of the sandbox you downloaded earlier,
Then enter the command ./sandbox up
to start the sandbox container and place it in Docker,
By default, the sandbox will be started with betanet support. To run the sandbox with support for another network enter ./sandbox up testnet
or ./sandbox up mainnet
.
Summary
With all the above steps completed, you have a ready-made environment to start working with the pyTeal library. You can now start writing your first smart contract, and deploy it on the network of your choice.
In the next article, we will introduce you to writing the simplest smart contract, along with deploying it on the Algorand network.