Introduction
It's difficult to create a chatbot that can have appropriate and realistic conversations. The GPT-2 model, which stands for Generative Pre-training Transformer 2, has been refined for conversational tasks after being trained on a vast amount of text data. In this post, we'll go through how to train a ChatGPT (Chat Generative Pre-training Transformer) model so that it may be adjusted to comprehend conversational cues and respond to them in a human-like manner. We'll go into detail about the crucial elements in this approach and how they help to produce a chatbot that can have conversations that flow naturally.
How ChatGPT was made?
ChatGPT is a variant of GPT (Generative Pre-training Transformer), which is a transformer-based language model developed by OpenAI. GPT was trained on a massive dataset of internet text and fine-tuned for specific tasks such as language translation and question answering. GPT-2, an advanced version of GPT, was trained on even more data and has the ability to generate human-like text. ChatGPT is fine-tuned version of GPT-2 to improve its performance in conversational AI tasks.
Training ChatGPT typically involves the following steps:
Collect a large dataset of conversational text, such as transcripts of customer service chats, social media conversations, or other forms of dialog.
What to bear in mind while doing this?
- The dataset should be large enough to capture a wide variety of conversational styles and topics. The more diverse the data, the better the model will be able to handle different types of input and generate more realistic and appropriate responses.
- The data should be representative of the types of conversations the model will be used for. For example, if the model will be used in a customer service chatbot, it should be trained on transcripts of customer service chats.
- If possible, include a variety of different speakers and languages. This will help the model to learn how to generate appropriate responses in different contexts and for different types of users.
- The data should be diverse in terms of the number of speakers, languages, accents, and cultural background.
- Label the data with the context of the conversation, such as topic, intent, sentiment, etc.
- Be sure to filter out any personal information, sensitive data, or any data that could be used to identify a person.
Preprocess the data to clean and format it for training the model. This may include tokenizing the text, removing special characters, and converting the text to lowercase.
A crucial part of training a conversational model like ChatGPT is preprocessing the data. It is beneficial to organize and clean the data so that the model can be trained with ease. Tokenization is the act of dividing the text into smaller parts, like words or phrases, in more detail. This assists in transforming the text into a format that the model can process more quickly. An application like NLTK or SpaCy can be used to perform the tokenization procedure.
Eliminating special characters and changing the text's case are further crucial steps. Converting the text to lowercase helps to standardize the data and lowers the amount of unique words the model needs to learn. Special characters can cause problems while training the model. In data preparation, it's also a good idea to eliminate stop words, which are frequent words like "a," "an," "the," etc. that don't have any significant meaning. It's also a good idea to replace dates or numbers with a specific token like "NUM" or "DATE" when preparing data. In data preparation, it's also a good idea to replace terms that are unknown or not in the model's lexicon with a unique token, such as "UNK."
It is crucial to note that preparing the data can take time, but it is necessary to make sure the model can benefit from the data. Preprocessing the data makes it easier for the model to interpret and learn from it. It also makes the data more consistent.
Fine-tune a pre-trained GPT-2 model on the conversational dataset using a framework such as Hugging Face's Transformers library.
The procedure entails tweaking the model's hyperparameters and running several epochs of training on the conversational dataset. This can be accomplished by utilizing a framework like Hugging Face's Transformers library, an open-source natural language processing toolkit that offers pre-trained models and user-friendly interfaces for optimizing them.
The rationale behind fine-tuning a pre-trained model is that it has previously been trained on a sizable dataset and has a solid grasp of the language's overall structure. The model can be refined on a conversational dataset so that it can learn to produce responses that are more tailored to the conversation's topic. The refined model will perform better at producing responses that are appropriate for customer service interactions, for instance, if the conversational dataset consists of transcripts of discussions with customer service representatives.
It is important to note that the model's hyperparameters, such as the learning rate, batch size, and number of layers, are frequently adjusted throughout the fine-tuning phase. The performance of the model can be significantly impacted by these hyperparameters, thus it's necessary to experiment with different settings to discover the ideal one. Additionally, depending on the size of the conversational dataset and the complexity of the model, the fine-tuning procedure can need a significant amount of time and processing resources. But in order for the model to understand the precise nuances and patterns of the dialogue and become more applicable to the task, this stage is essential.
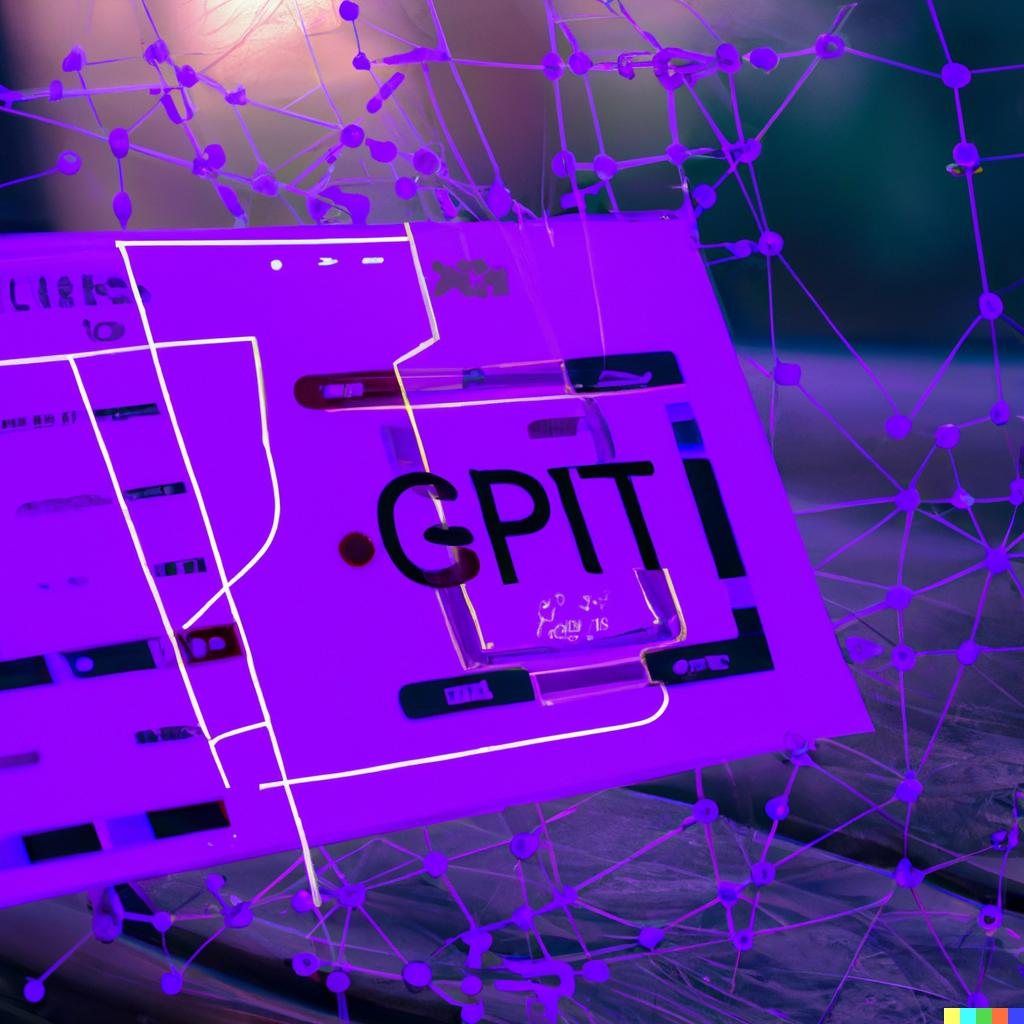
Evaluate the model's performance on a held-out test set to ensure it generates realistic and appropriate responses.
A held-out test set, which is a dataset distinct from the data used to train and fine-tune the model, is one popular strategy. The model's capacity to produce realistic and pertinent responses is evaluated using the held-out test set.
Measuring a conversational model's capacity to provide suitable and realistic responses is a typical technique to assess its performance. This can be achieved by assessing the similarity between the model-generated and human-written responses. Utilizing metrics like BLEU, METEOR, ROUGE, and others is one approach to do this. These metrics assess how comparable the automatically generated and manually written responses are to one another.
Measuring a conversational model's capacity to comprehend and respond to various inputs is another technique to assess its performance. This can be accomplished by putting the model to the test with various inputs and evaluating how well it responds to them. You might test the model using inputs with various intents, subjects, or feelings and assess how effectively it can react.
Use the trained model to generate responses to new input.
Once trained and improved, the model can be utilized to produce answers to fresh input. The last stage in creating a chatbot is testing the model to make sure it can respond realistically and appropriately to new input. The trained model processes the input before producing a response. It's crucial to remember that the caliber of the reaction will depend on the caliber of the training data and the procedure of fine-tuning.
Context is crucial when using a trained model to generate responses in a conversation. To produce responses that are relevant and appropriate to the current conversation, it's important to keep track of the conversation history. A dialogue manager, which manages the conversation history and creates suitable inputs for the model, can be used to accomplish this.
Especially when employing a trained model to generate responses, it's critical to ensure the quality of the responses the model generates. As the model might not always create suitable or realistic responses, a technique for weeding out improper responses should be in place. Using a post-processing phase that would filter out inappropriate responses and choose the best one is one way to accomplish this.
Conclusion
Training a ChatGPT model is a multi-step process that requires a large amount of data. The GPT-2 model with its ability to generate human-like text and fine-tuning it with conversational dataset can lead to very powerful results which might be extremely helpful in everyday life. The process of training is essential in creating a chatbot that can understand and respond to conversational prompts in a natural and seamless manner. As the field of AI continues to evolve, the development of sophisticated chatbots will play an increasingly important role in enhancing the way we interact with technology. Interested? Check out our other articles related to AI!