Introduction
In the ever-evolving world of software development, it has become increasingly important to ensure that software products are reliable, scalable, and efficient. One of the key components of software development is testing, which involves checking for defects and ensuring that the software meets the required specifications. With the increasing complexity of software, it has become more challenging to manually test software products. AI-powered testing tools have emerged as a solution to this problem. In this article, we will explore some of the top AI-powered testing tools that are changing the landscape of software testing.
1. Test Automation Tools
By automating the execution of test cases, test automation solutions reduce down on the time and labor needed for manual testing. Machine learning techniques are used by AI-powered test automation technologies to learn from prior test runs and improve test performance. Regression testing can be automated with the use of these tools, freeing up testers to work on more difficult jobs. By running a large number of test cases quickly—something manual testing cannot do—they can also aid in enhancing test coverage.
The earlier errors are found in the software development lifecycle, the quicker and less expensive it is to rectify them. This is another benefit of AI-powered test automation technologies. They can also offer insightful information about the functionality and behavior of the product, assisting in pinpointing areas that could use improvement.
Examples of tools:
- Testim: https://www.testim.io/
- Applitools: https://applitools.com/
2. Intelligent Test Data Management
The process of creating, storing, and maintaining test data, which is necessary for writing and running test cases, is known as intelligent test data management. The generation of appropriate test data that truly depicts the behavior of the software, however, can be time-consuming and difficult. In order to solve this problem, AI-powered test data management systems use machine learning algorithms to evaluate the behavior of the program and provide test data that simulates real-world scenarios, hence enhancing the quality of test cases and assuring better coverage of test scenarios. By reducing the time and effort required to create and manage test data, these tools help in optimizing the testing process. Additionally, they help identify data dependencies and relationships, ensuring that the test data accurately reflects the software's behavior, while also ensuring data privacy and security by masking sensitive data and complying with data protection regulations.
Examples of tools:
- GenRocket: https://www.genrocket.com/
- QuerySurge: https://www.querysurge.com/
3. Intelligent Test Generation
Intelligent test generation technologies examine code changes or business requirements and automatically produce test cases using machine learning methods. In complicated software projects, where writing test cases can be labor-intensive and error-prone, this is especially advantageous. These technologies enhance testing quality while reducing the time and effort needed to generate test cases by automating the test generation process. The generated test cases ensure higher test coverage by covering the most important scenarios and detecting edge cases and scenarios that may be challenging for human testers to uncover. Also, automating the test creation process allows testers to concentrate on testing jobs that are more complicated, resulting in more effective testing procedures and higher-quality software products.
Examples of tools:
- Diffblue: https://www.diffblue.com/
- Test.ai: https://test.ai/
4. Defect Prediction and Analysis
AI may replicate actual situations and create load on the system to gauge its performance, dependability, and scalability. These AI-powered performance testing tools can assist in locating performance snags and other problems that may have an influence on the user experience. They evaluate the system's behavior under stress using machine learning techniques to find patterns that can improve the system's performance. These technologies can provide precise and trustworthy insights into the system's performance by replicating real-world events. This enables teams to find and fix performance problems before they have an impact on users.
Examples of tools:
- DeepCode: https://www.deepcode.ai/
- CodeScene: https://codescene.io/
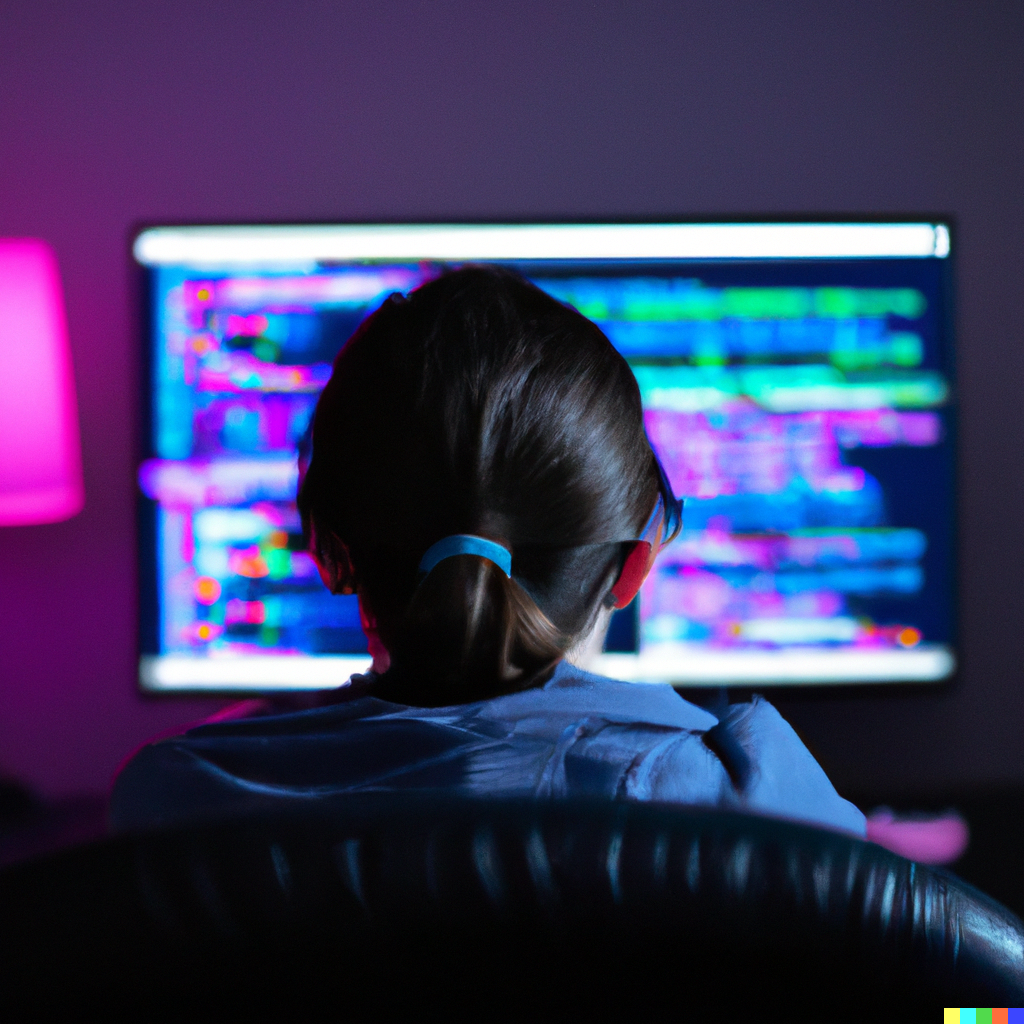
5. Performance Testing
AI can produce loads on the system to test its performance, dependability, and scalability by simulating real-world events. The user experience can be negatively impacted by performance bottlenecks and other problems, which can be found with these AI-powered performance testing tools. They examine the system's performance under load using machine learning methods to look for patterns that might be improved. These technologies may simulate real-world events and offer precise and trustworthy insights into the system's performance, allowing teams to find and fix performance problems before they have an impact on consumers.
Examples of tools:
- BlazeMeter: https://www.blazemeter.com/
- Gatling: https://gatling.io/
6.Intelligent Test Reporting
AI-powered test reporting systems can generate results that are simple to read and understand while also automating the reporting process, giving users important insights about the software's quality and the efficiency of the testing process. These reports offer real-time insights into the testing process and may be used to spot patterns and trends in defects, test coverage, and other metrics. This allows teams to make data-driven decisions and find problems as soon as possible. These tools can assist in streamlining the testing process and enhancing the overall quality of the software by saving time and enhancing the accuracy and efficacy of the reporting process.Teams can have a deeper understanding of the insights provided by the reports and take the necessary action with the use of natural language processing and other machine learning techniques employed in these products.
Examples of tools:
- Mabl: https://www.mabl.com/
- TestFLO for Jira: https://www.deviniti.com/apps/testflo-for-jira
Conclusion
The way we approach software testing has changed as a result of AI-powered testing tools. They have greatly lowered the amount of time and effort needed for testing while simultaneously raising the standard of testing. These tools may imitate real-world situations, produce test cases, spot performance bottlenecks, and offer insightful information about the testing procedure, all of which help produce higher-quality software. Software development teams can save time, cut expenses, and increase the dependability and scalability of their software products by utilizing these tools. A critical step in ensuring that software development keeps up with the needs of the current world is the introduction of AI-powered testing technologies.
Also, don't miss these free AI tools for developers!