As the web3 field grows in complexity, traditional analytical tools often fall short in capturing the dynamics of digital markets. This is where Monte Carlo simulations come into play, offering a mathematical technique to model systems fraught with uncertainty.
Monte Carlo simulations employ random sampling to understand probable outcomes in processes that are too complex for straightforward analytic solutions. By simulating thousands, or even millions, of scenarios, Monte Carlo methods can provide insights into the likelihood of different outcomes, helping stakeholders make informed decisions under conditions of uncertainty.
In this article, we will explore the role of Monte Carlo simulations within the context of tokenomics. illustrating how they are employed to forecast market dynamics, assess risk, and optimize strategies in the volatile realm of cryptocurrencies. By integrating this powerful tool, businesses and investors can enhance their analytical capabilities, paving the way for more resilient and adaptable economic models in the digital age.
Understanding Monte Carlo Simulations
The Monte Carlo method is an approach to solving problems that involve random sampling to understand probable outcomes. This technique was first developed in the 1940s by scientists working on the atomic bomb during the Manhattan Project. The method was designed to simplify the complex simulations of neutron diffusion, but it has since evolved to address a broad spectrum of problems across various fields including finance, engineering, and research.
Random Sampling and Statistical Experimentation
At the heart of Monte Carlo simulations is the concept of random sampling from a probability distribution to compute results. This method does not seek a singular precise answer but rather a probability distribution of possible outcomes. By performing a large number of trials with random variables, these simulations mimic the real-life fluctuations and uncertainties inherent in complex systems.
Role of Randomness and Probability Distributions in Simulations
Monte Carlo simulations leverage the power of probability distributions to model potential scenarios in processes where exact outcomes cannot be determined due to uncertainty. Each simulation iteration uses randomly generated values that follow a specific statistical distribution to model different outcomes. This method allows analysts to quantify and visualize the probability of different scenarios occurring.
The strength of Monte Carlo simulations lies in the insight they offer into potential risks. They allow modelers to see into the probabilistic "what-if" scenarios that more closely mimic real-world conditions.
Monte Carlo Simulations in Tokenomics
Monte Carlo simulations are instrumental tool for token engineers. They're so useful due to their ability to model emergent behaviors. Here are some key areas where these simulations are applied:
Pricing and Valuation of Tokens
Determining the value of a new token can be challenging due to the volatile nature of cryptocurrency markets. Monte Carlo simulations help by modeling various market scenarios and price fluctuations over time, allowing analysts to estimate a token's potential future value under different conditions.
Assessing Market Dynamics and Investor Behavior
Cryptocurrency markets are influenced by a myriad of factors including regulatory changes, technological advancements, and shifts in investor sentiment. Monte Carlo methods allow researchers to simulate these variables in an integrated environment to see how they might impact token economics, from overall market cap fluctuations to liquidity concerns.
Assesing Possible Risks
By running a large number of simulations it’s possible to stress-test the project in multiple scenarios and identify emergent risks. This is perhaps the most important function of Monte Carlo Process, since these risks can’t be assessed any other way.
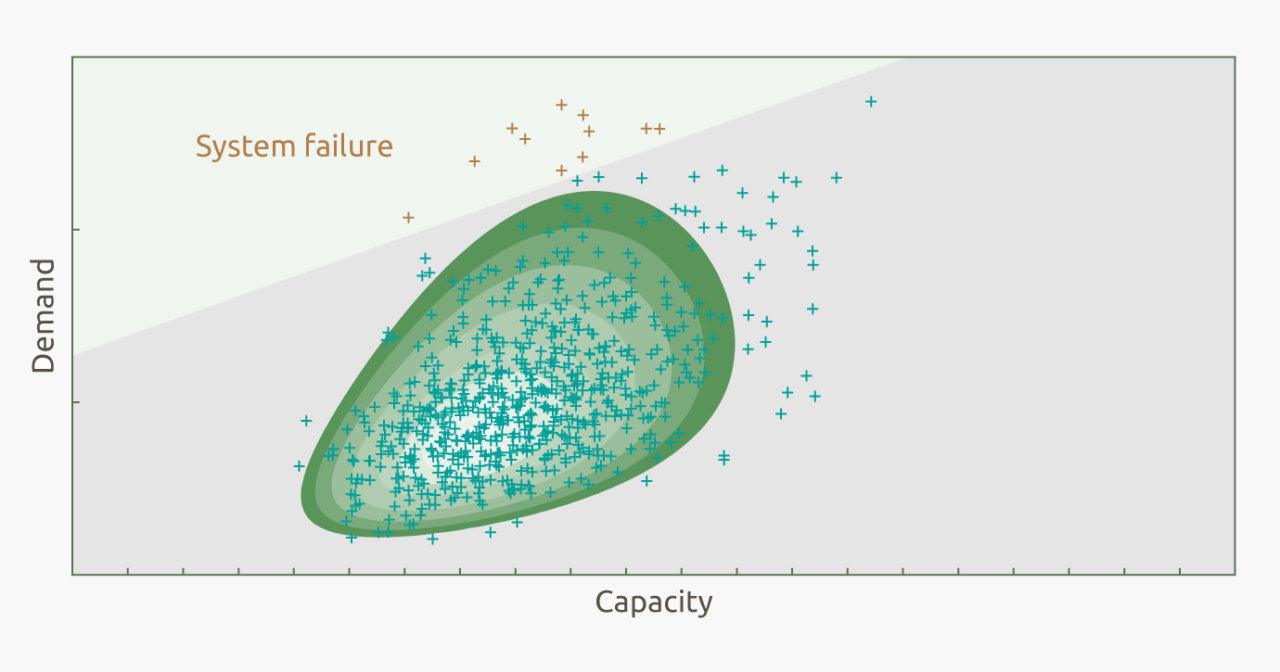
Benefits of Using Monte Carlo Simulations
By generating a range of possible outcomes and their probabilities, Monte Carlo simulations help decision-makers in the cryptocurrency space anticipate potential futures and make informed strategic choices. This capability is invaluable for planning token launches, managing supply mechanisms, and designing marketing strategies to optimize market penetration.
Using Monte Carlo simulations, stakeholders in the tokenomics field can not only understand and mitigate risks but also explore the potential impact of different strategic decisions. This predictive power supports more robust economic models and can lead to more stable and successful token launches.
Implementing Monte Carlo Simulations
Several tools and software packages can facilitate the implementation of Monte Carlo simulations in tokenomics. One of the most notable is cadCAD, a Python library that provides a flexible and powerful environment for simulating complex systems.
Overview of cadCAD configuration Components
To better understand how Monte Carlo simulations work in practice, let’s take a look at the cadCAD code snippet:
sim_config = {
'T': range(200), # number of timesteps
'N': 3, # number of Monte Carlo runs
'M': params # model parameters
}
Explanation of Simulation Configuration Components
T: Number of Time Steps
- Definition: The 'T' parameter in CadCAD configurations specifies the number of time steps the simulation should execute. Each time step represents one iteration of the model, during which the system is updated. That update is based on various rules defined by token engineers in other parts of the code. For example: we might assume that one iteration = one day, and define data-based functions that predict token demand on that day.
N: Number of Monte Carlo Runs
- Definition: The 'N' parameter sets the number of Monte Carlo runs. Each run represents a complete execution of the simulation from start to finish, using potentially different random seeds for each run. This is essential for capturing variability and understanding the distribution of possible outcomes. For example, we can acknowledge that token’s price will be correlated with the broad cryptocurrency market, which acts somewhat unpredictably.
M: Model Parameters
- Definition: The 'M' key contains the model parameters, which are variables that influence system's behavior but do not change dynamically with each time step. These parameters can be constants or distributions that are used within the policy and update functions to model the external and internal factors affecting the system.
Importance of These Components
Together, these components define the skeleton of your Monte Carlo simulation in CadCAD. The combination of multiple time steps and Monte Carlo runs allows for a comprehensive exploration of the stochastic nature of the modeled system. By varying the number of timesteps (T) and runs (N), you can adjust the depth and breadth of the exploration, respectively. The parameters (M) provide the necessary context and ensure that each simulation is realistic.
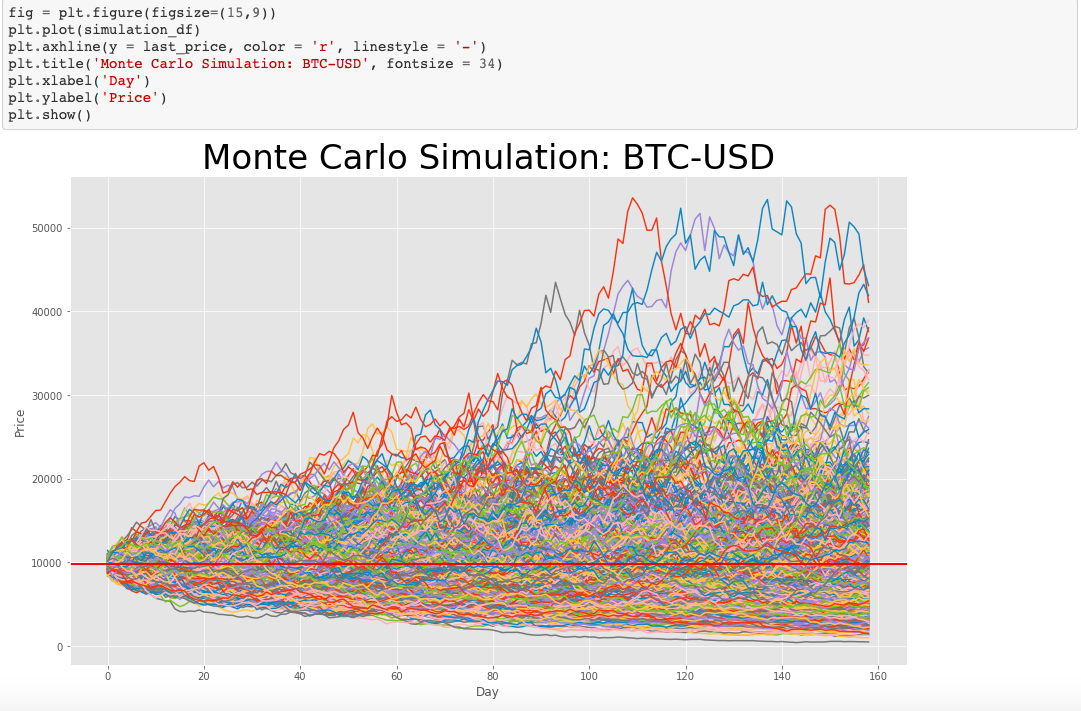
Conclusion
Monte Carlo simulations represent a powerful analytical tool in the arsenal of token engineers. By leveraging the principles of statistics, these simulations provide deep insights into the complex dynamics of token-based systems. This method allows for a nuanced understanding of potential future scenarios and helps with making informed decisions.
We encourage all stakeholders in the blockchain and cryptocurrency space to consider implementing Monte Carlo simulations. The insights gained from such analytical techniques can lead to more effective and resilient economic models, paving the way for the sustainable growth and success of digital currencies.
If you're looking to create a robust tokenomics model and go through institutional-grade testing please reach out to contact@nextrope.com. Our team is ready to help you with the token engineering process and ensure your project’s resilience in the long term.
FAQ
What is a Monte Carlo simulation in tokenomics context?
- It's a mathematical method that uses random sampling to predict uncertain outcomes.
What are the benefits of using Monte Carlo simulations in tokenomics?
- These simulations help foresee potential market scenarios, aiding in strategic planning and risk management for token launches.
Why are Monte Carlo simulations unique in cryptocurrency analysis?
- They provide probabilistic outcomes rather than fixed predictions, effectively simulating real-world market variability and risk.